Unraveling the Mysteries of Data Science: A Guide to the Data Analytics and Machine Learning Workflow
- paixomaik6
- 20 de dez. de 2023
- 2 min de leitura
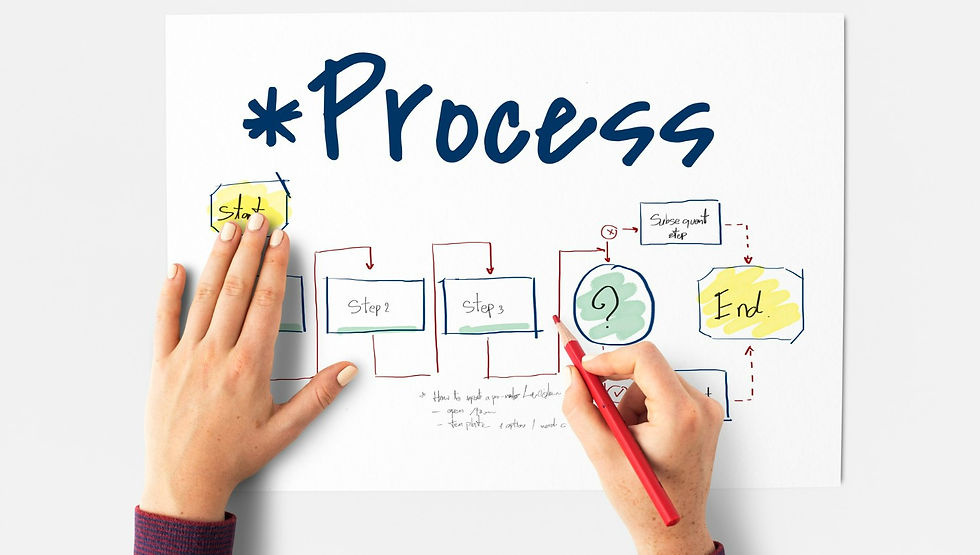
In the ever-evolving landscape of data science, understanding the intricate processes that drive this field is crucial. Today, I want to delve into what is popularly known as the "Data Analytics and Machine Learning Workflow," or in broader terms, the "Data Science Workflow."
This comprehensive guide aims to demystify the sequence of steps and the synergy between different professionals in a typical data-driven project.
The Collaborative Symphony of Professionals
The data science workflow is a testament to the power of collaboration. It brings together three pivotal roles:
Data Engineers: These are the architects who construct the infrastructure for data generation, storage, and retrieval. They lay the foundation upon which data operations are built, ensuring the availability, integrity, and efficiency of data systems.
Data Analysts: With a keen eye for detail, data analysts scrutinize the gathered data. They are the detectives who interpret data, identify trends, and provide actionable insights. Their analyses form the backbone of data-driven decision-making in organizations.
Data Scientists: These individuals are the alchemists of the data world. They apply advanced techniques, including machine learning and statistical modeling, to extract deeper insights and predictions from data. Their work often dictates strategic directions and innovative solutions.
The Stages of the Workflow
The Data Science Workflow is an iterative process that continuously evolves. Let's break it down:
Data Collection: The journey begins with gathering relevant data. This stage is about identifying and extracting useful data from various sources.
Preprocessing: Raw data is rarely perfect. Preprocessing involves cleaning and structuring data to make it suitable for analysis.
Analysis: Here, data is examined to uncover patterns and relationships. This stage often involves exploratory data analysis and hypothesis testing.
Modeling: The crux of predictive analytics. This phase involves creating algorithms and statistical models that can predict trends or outcomes.
Deployment: Developed models are put into practice. This could be in the form of a data product or integrating the model into existing systems.
Monitoring: Post-deployment, it’s vital to monitor the performance of models, ensuring they function as intended.
Iterative Refinement: Data science is never static. Models and strategies are continuously refined and improved based on new data and insights.
The Impact and Future of Data Science
As we advance, the significance of this workflow only grows. It's not just about handling data; it's about turning data into a strategic asset. This workflow is at the heart of transforming raw data into actionable insights, driving innovation, and shaping the future of businesses and technologies.
The data science workflow represents a journey of discovery, innovation, and continuous improvement. It's a dynamic process that adapts and evolves, much like the field of data science itself. As we embrace new technologies and methodologies, this workflow will undoubtedly evolve, further enhancing our ability to harness the power of data.
Hi, I’m Maik. Hope you enjoyed the article. If you have any questions or want to connect with me and access more content, follow my channels:
LinkedIn: https://www.linkedin.com/in/maikpaixao/
Twitter: https://twitter.com/maikpaixao
Facebook: https://www.facebook.com/maikpaixao
Youtube: https://www.youtube.com/@maikpaixao
Instagram: https://www.instagram.com/datamaikpaixao/
Github: https://github.com/maikpaixao
Comments